Introduction
The integration of artificial intelligence (AI) in modern research has been nothing short of transformative. AI tools for research improve the way we collect and interpret data, unlocking new avenues of discovery and innovation.
However, AI is not a one-size-fits-all solution. UX designers must approach this technology with a nuanced understanding of its capabilities and limitations.
This article will consider the opportunities and challenges of augmenting human researchers with AI tools. We will explore the ways AI can enhance research, while also examining the potential pitfalls that must be navigated in the process. Read on to learn more!
Why We Need AI in Research
The use of AI in research ushered in a new era of efficiency and innovation. With these tools, we are experiencing a degree of productivity that was otherwise impossible.
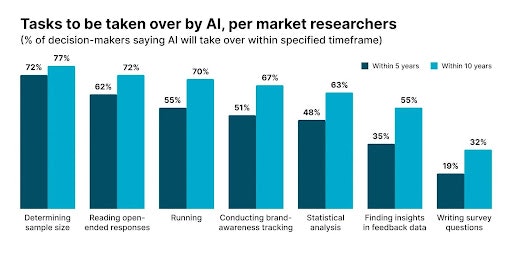
Below, let's consider the specific reasons why AI is a valuable asset in modern research:
1. Accelerate Discovery
AI tools are particularly efficient in idea discovery. Here's how they can accelerate the process:
Hypothesis Generation: AI can generate new hypotheses based on existing data, stimulating novel research directions.
Data Exploration: With AI, it's easy to uncover hidden patterns and relationships within large and complex datasets that may not be apparent to human researchers.
Problem Identification: AI does well in identifying emerging trends and challenges that may require further investigation.
2. Enhance Collaboration
Gone are the days when collaboration between different research teams was a hassle. AI offers features that include:
Data Sharing: AI can facilitate the sharing and collaboration of data across research teams and institutions.
Knowledge Synthesis: AI tools are capable of synthesizing information from diverse sources, providing researchers with a comprehensive understanding of a particular topic.
Cross-Disciplinary Research: AI can bridge gaps between different fields of study, promoting interdisciplinary collaboration.
3. Improve Decision-Making
Improved decision-making has been one of the most notable contributions of AI. With these tools, you can make:
Evidence-Based Decisions: AI can provide researchers with evidence-based recommendations, supporting informed decision-making.
Risk Assessment: AI tools help researchers assess the potential risks and benefits of different research approaches.
Resource Allocation: AI can optimize the allocation of resources, ensuring that research efforts are focused on the most promising areas.
Limitations of AI in Research
Now that we have covered the numerous benefits of AI-powered research, it is important to acknowledge its limitations. These limitations can arise from the inherent nature of AI algorithms, the quality of the data used to train them, and the ethical implications of their use.
The Black Box Problem
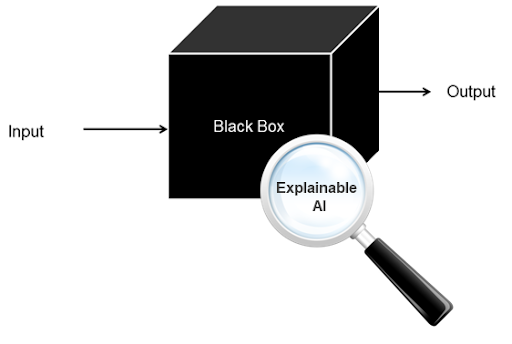
One of the major challenges associated with AI is the "black box" problem. This refers to the difficulty of understanding how AI algorithms arrive at their decisions.
Many AI models are complex and opaque by design, making it difficult to explain their reasoning to non-experts. This can be problematic in research, where it is often important to understand the underlying logic behind a particular result.
Data Quality and Bias
The quality of the data used to train AI models can significantly impact their performance. If the data is biased or low-quality, the AI may produce biased or inaccurate results. This can lead to misleading conclusions and undermine the credibility of research. Let's illustrate this with an example.
Scenario:
Imagine a research team developing an AI model to predict the likelihood of a student's success in a particular college program. The dataset used to train the model is primarily composed of data from students from affluent backgrounds. As a result, the model is biased towards students from affluent backgrounds and may underpredict the success of students from disadvantaged backgrounds.
This could lead to discriminatory outcomes, such as denying admissions to qualified students from disadvantaged backgrounds or allocating resources unfairly.
Ethical Considerations
The use of AI in research raises a number of ethical concerns. These include privacy, accountability, and fairness.
Privacy
To function properly, AI needs to collect and process large amounts of personal data. This raises concerns about privacy and data security. Researchers must ensure that they obtain informed consent from participants and implement appropriate data protection measures.
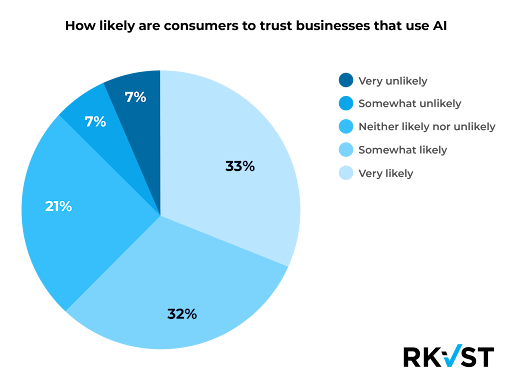
Accountability
It can be difficult to hold AI systems accountable for their actions. If an AI system makes a wrong or harmful decision, it may be unclear who is responsible. For instance, if AI infringes on a creator's copyright, it's difficult to hold someone accountable.
Fairness
AI systems can perpetuate or amplify existing biases, leading to unfair or discriminatory outcomes. This is why researchers must take steps to ensure that AI is used fairly and equitably.
The Importance of Awareness
Addressing these limitations requires a careful and thoughtful approach. Researchers must be aware of the potential pitfalls of AI and take steps to mitigate them. By doing so, we can utilize AI technology to its fullest strength while ensuring that it's responsible and ethical.
Successful AI Applications in Research
AI has been successfully applied in a wide range of research fields, demonstrating its potential to enhance efficiency and accuracy. This section will present several case studies showcasing the successful integration of AI in different industries. We also highlight the takeaways from these successful projects.
Examples of AI-Augmented Research Projects and Tools
UX Pilot
UX Pilot is a comprehensive user experience design tool. It relies on AI to facilitate and improve different aspects of the design process, including user and market research. For instance, UX designers utilize this tool to quickly synthesize data from preliminary user research and discover new insights.
AlphaFold
This AI system from Google's DeepMind has revolutionized protein structure prediction, accelerating drug discovery and understanding of biological processes. By accurately predicting protein structures, AlphaFold is significantly advancing research in fields such as medicine and biotechnology.
IBM Watson
IBM Watson has been used in various research fields, such as healthcare and genomics, to analyze vast amounts of data and identify patterns that would be difficult for humans to detect. For example, Watson has been used to assist doctors in diagnosing diseases and developing personalized treatment plans.
Natural Language Processing (NLP) Tools
NLP tools are becoming popular in fields like linguistics and literature. This is because they can process text data and extract key information. For instance, NLP tools like GPT can analyze documents, identify themes and patterns, and gain insights into events.
Lessons Learned
The surge of AI usage across many industries and the resulting business impact has taught us many lessons.
Data Quality is Crucial: The quality of the data used to train AI models is essential for accurate and reliable results. Researchers must ensure that their data is clean, representative, and free from bias.
Human Oversight is Necessary: While AI can automate many tasks, human oversight is still required to ensure that AI is used ethically and effectively. Humans can provide context, interpretation, and judgment that AI may lack.
Collaboration is Key: Successful AI-augmented research often involves collaboration between researchers, data scientists, and AI experts. By working together, these individuals can leverage their respective expertise to achieve better outcomes.
Ethical Usage: Ethical considerations, such as privacy and bias, must be carefully addressed when using AI in research. Researchers must ensure that AI is used in a responsible and equitable manner.
The Future of AI in Research
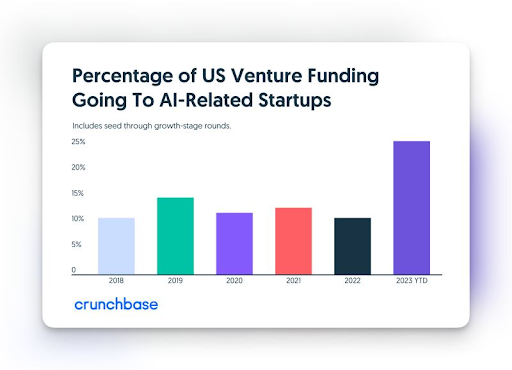
With AI-related startups on the rise, we can expect to see more adoption.
Let's explore some of the emerging trends and technologies in AI research applications.
Emerging Trends and Technologies:
Generative AI: Generative AI models, such as GPT-4, are capable of generating human-quality text, images, and code. These models have the potential to improve research by automating tasks like literature reviews and data analysis.
AI In UX Design: AI UX design tools can quickly generate prototypes and user research material for interviews or usability studies. This can help designers optimize their workflows.
Explainable AI: Explainable AI (XAI) aims to make AI models more transparent and understandable. This is particularly important in research, where it is often necessary to explain the reasoning behind AI-generated results.
AI for Scientific Discovery: AI is being used to discover new scientific knowledge, such as identifying new drug candidates or predicting the properties of materials.
Potential Challenges and Opportunities
As AI continues to advance, it is important to be aware of the potential challenges and opportunities that may arise. Some of these include:
Ethical Concerns: The use of AI in research raises ethical concerns, such as bias, privacy, and accountability.
Job Displacement: AI could potentially lead to job displacement for human researchers.
Overreliance on AI: Overreliance on AI could lead to a decline in human expertise and judgment.
However, AI also presents significant opportunities. It can accelerate research, improve accuracy, and enable new forms of scientific discovery.
The Role of Human Researchers
Despite the advancements in AI, human researchers will continue to play a vital role in the research process. Humans can provide context, interpretation, and judgment that AI often lacks. They can also ensure that AI is used ethically and responsibly.
In the future, human researchers will likely work in close collaboration with AI tools, leveraging their respective strengths. This mutual relationship will enable researchers to achieve new heights of discovery and accuracy.
Conclusion
The integration of AI into research offers numerous benefits as we have seen. AI can automate tasks, analyze large datasets, and generate new insights, freeing up human researchers to focus on higher-level activities.
However, we must recognize the limitations such as the black box problem, data quality issues, and ethical concerns. Researchers should explore the potential of AI to enhance their work while remaining mindful of these limitations.
Ultimately, the most successful research will involve a mutual relationship between human researchers and AI tools. Humans can provide context and the much-needed ethical oversight, while AI can streamline research processes.
FAQs
How can researchers ensure that AI tools are used ethically in research?
Researchers can ensure ethical AI use by conducting thorough ethical assessments, involving diverse stakeholders, and regularly monitoring and evaluating AI systems. Additionally, adhering to relevant ethical guidelines and regulations is crucial.
What are the potential risks of overreliance on AI in research?
Overreliance on AI can lead to a decline in human expertise, judgment, and creativity. It can also increase the risk of bias and errors if AI systems are not used appropriately. Human oversight and critical thinking remain essential for robust research.
How can researchers effectively integrate AI tools into their research workflows?
Successful integration of AI involves careful planning, training, and collaboration. Researchers should identify suitable AI tools, ensure data quality, and develop clear guidelines for AI use. Additionally, ongoing evaluation and adaptation are essential to maximize the benefits of AI.